
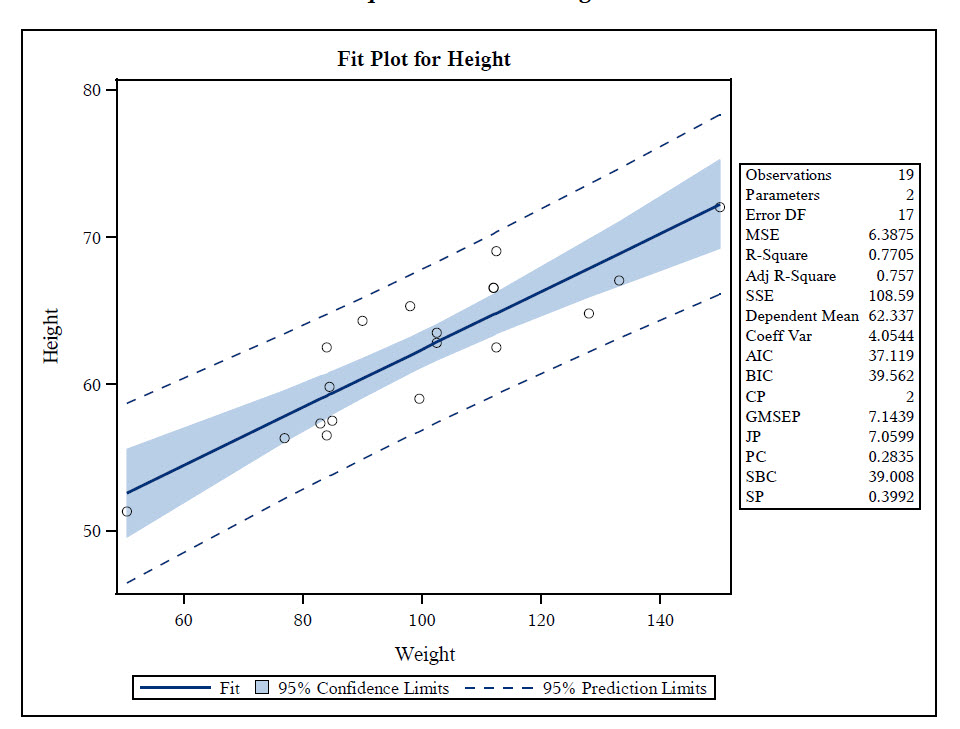
Let’s take a look at the first type of plot: The diagnostic plots show residuals in four different ways. They are extreme values based on each criterion and identified by the row numbers in the data set.

You will often see numbers next to some points in each plot. Par(mfrow=c(2,2)) # Change the panel layout to 2 x 2 Then R will show you four diagnostic plots one by one. It’s very easy to run: just use a plot() to an lm object after running an analysis. In this post, I’ll walk you through built-in diagnostic plots for linear regression analysis in R (there are many other ways to explore data and diagnose linear models other than the built-in base R function though!). Using this information, not only could you check if linear regression assumptions are met, but you could improve your model in an exploratory way. Residuals are leftover of the outcome variable after fitting a model (predictors) to data and they could reveal unexplained patterns in the data by the fitted model. Residuals could show how poorly a model represents data. We pay great attention to regression results, such as slope coefficients, p-values, or R 2 that tell us how well a model represents given data. We can check if a model works well for data in many different ways. After running a regression analysis, you should check if the model works well for data. You might think that you’re done with analysis.
#SAS OBJECT FITPLOT NOT CREATED SOFTWARE#
You ran a linear regression analysis and the stats software spit out a bunch of numbers.
